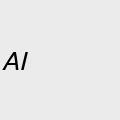
2024-03-13 06:46:46
Transforming Competition into Collaboration: The Revolutionary Role of Multi-Agent Systems and Language Models in Modern Organizations
Carlos Jose Xavier Cruz
https://arxiv.org/abs/2403.07769
Transforming Competition into Collaboration: The Revolutionary Role of Multi-Agent Systems and Language Models in Modern Organizations
Carlos Jose Xavier Cruz
https://arxiv.org/abs/2403.07769
This https://arxiv.org/abs/2402.11550 has been replaced.
initial toot: https://mastoxiv.page/@arXiv_csCL_…
Transforming Competition into Collaboration: The Revolutionary Role of Multi-Agent Systems and Language Models in Modern Organizations
Carlos Jose Xavier Cruz
https://arxiv.org/abs/2403.07769
Simulating Human Strategic Behavior: Comparing Single and Multi-agent LLMs
Karthik Sreedhar, Lydia Chilton
https://arxiv.org/abs/2402.08189 https://…
This https://arxiv.org/abs/2311.09618 has been replaced.
initial toot: https://mastoxiv.page/@arX…
This https://arxiv.org/abs/2402.11550 has been replaced.
initial toot: https://mastoxiv.page/@arXiv_csCL_…
This https://arxiv.org/abs/2309.07055 has been replaced.
initial toot: https://mastoxiv.page/@arXiv_csCY_…
This https://arxiv.org/abs/2403.03434 has been replaced.
initial toot: https://mastoxiv.page/@arXiv_csMA_…
LLM-Based Multi-Agent Systems for Software Engineering: Vision and the Road Ahead
Junda He, Christoph Treude, David Lo
https://arxiv.org/abs/2404.04834 htt…
This https://arxiv.org/abs/2308.11959 has been replaced.
initial toot: https://mastoxiv.page/@arXiv_ees…
Variational Inference of Parameters in Opinion Dynamics Models
Jacopo Lenti, Fabrizio Silvestri, Gianmarco De Francisci Morales
https://arxiv.org/abs/2403.05358
A Guide to Re-Implementing Agent-based Models: Experiences from the HUMAT Model
\"Onder G\"urcan, Timo Szczepanska, Patrycja Antosz
https://arxiv.org/abs/2405.03994
A Model-based Multi-Agent Personalized Short-Video Recommender System
Peilun Zhou, Xiaoxiao Xu, Lantao Hu, Han Li, Peng Jiang
https://arxiv.org/abs/2405.01847
Multi-Agent RL-Based Industrial AIGC Service Offloading over Wireless Edge Networks
Siyuan Li, Xi Lin, Hansong Xu, Kun Hua, Xiaomin Jin, Gaolei Li, Jianhua Li
https://arxiv.org/abs/2405.02972
A Guide to Re-Implementing Agent-based Models: Experiences from the HUMAT Model
\"Onder G\"urcan, Timo Szczepanska, Patrycja Antosz
https://arxiv.org/abs/2405.03994
Partner selection and evolution of out-group avoidance
Hirofumi Takesue
https://arxiv.org/abs/2403.06893 https://arxiv.org/pdf/2403.0…
This https://arxiv.org/abs/2402.17785 has been replaced.
initial toot: https://mastoxiv.page/@arXiv_csSD_…
This https://arxiv.org/abs/2404.10861 has been replaced.
initial toot: https://mastoxiv.page/@arXiv_csNE_…
This https://arxiv.org/abs/2404.18704 has been replaced.
initial toot: https://mastoxiv.page/@arXiv_mat…
The importance of the incubation time distribution in compartmental epidemiological models
Eric Rozan, Marcelo N Kuperman, Sebastian Bouzat
https://arxiv.org/abs/2403.04505
Dynamic Human Trust Modeling of Autonomous Agents With Varying Capability and Strategy
Jason Dekarske (University of California, Davis), Zhaodan Kong (University of California, Davis), Sanjay Joshi (University of California, Davis)
https://arxiv.org/abs/2404.19291 https://arxiv.org/pdf/2404.19291
arXiv:2404.19291v1 Announce Type: new
Abstract: Objective We model the dynamic trust of human subjects in a human-autonomy-teaming screen-based task.
Background Trust is an emerging area of study in human-robot collaboration. Many studies have looked at the issue of robot performance as a sole predictor of human trust, but this could underestimate the complexity of the interaction.
Method Subjects were paired with autonomous agents to search an on-screen grid to determine the number of outlier objects. In each trial, a different autonomous agent with a preassigned capability used one of three search strategies and then reported the number of outliers it found as a fraction of its capability. Then, the subject reported their total outlier estimate. Human subjects then evaluated statements about the agent's behavior, reliability, and their trust in the agent.
Results 80 subjects were recruited. Self-reported trust was modeled using Ordinary Least Squares, but the group that interacted with varying capability agents on a short time order produced a better performing ARIMAX model. Models were cross-validated between groups and found a moderate improvement in the next trial trust prediction.
Conclusion A time series modeling approach reveals the effects of temporal ordering of agent performance on estimated trust. Recency bias may affect how subjects weigh the contribution of strategy or capability to trust. Understanding the connections between agent behavior, agent performance, and human trust is crucial to improving human-robot collaborative tasks.
Application The modeling approach in this study demonstrates the need to represent autonomous agent characteristics over time to capture changes in human trust.
Control of high-dimensional collective dynamics by deep neural feedback laws and kinetic modelling
Giacomo Albi, Sara Bicego, Dante Kalise
https://arxiv.org/abs/2404.02825
CACTUS: Chemistry Agent Connecting Tool-Usage to Science
Andrew D. McNaughton, Gautham Ramalaxmi, Agustin Kruel, Carter R. Knutson, Rohith A. Varikoti, Neeraj Kumar
https://arxiv.org/abs/2405.00972
A Unified Debugging Approach via LLM-Based Multi-Agent Synergy
Cheryl Lee, Chunqiu Steven Xia, Jen-tse Huang, Zhouruixin Zhu, Lingming Zhang, Michael R. Lyu
https://arxiv.org/abs/2404.17153
A Pontryagin Maximum Principle for agent-based models with convex state space
Stefano Almi, Riccardo Durastanti, Francesco Solombrino
https://arxiv.org/abs/2402.13680
Go Beyond Black-box Policies: Rethinking the Design of Learning Agent for Interpretable and Verifiable HVAC Control
Zhiyu An, Xianzhong Ding, Wan Du
https://arxiv.org/abs/2403.00172
Analysis of Phylogeny Tracking Algorithms for Serial and Multiprocess Applications
Matthew Andres Moreno, Santiago Rodriguez Papa, Emily Dolson
https://arxiv.org/abs/2403.00246
An AI-enabled Agent-Based Model and Its Application in Measles Outbreak Simulation for New Zealand
Sijin Zhang, Alvaro Orsi, Richard Dean, Lei Chen, Rachel Qiu, Jiawei Zhao
https://arxiv.org/abs/2403.03434
This https://arxiv.org/abs/2312.15910 has been replaced.
link: https://scholar.google.com/scholar?q=a
A Belief Propagation Algorithm for Multipath-based SLAM with Multiple Map Features: A mmWave MIMO Application
Xuhong Li, Xuesong Cai, Erik Leitinger, Fredrik Tufvesson
https://arxiv.org/abs/2403.10095
ByteComposer: a Human-like Melody Composition Method based on Language Model Agent
Xia Liang, Jiaju Lin, Xinjian Du
https://arxiv.org/abs/2402.17785 https:…
Modelling crypto markets by multi-agent reinforcement learning
Johann Lussange, Stefano Vrizzi, Stefano Palminteri, Boris Gutkin
https://arxiv.org/abs/2402.10803
This https://arxiv.org/abs/2312.09007 has been replaced.
initial toot: https://mastoxiv.page/@arXiv_csIT_…
MAGIS: LLM-Based Multi-Agent Framework for GitHub Issue Resolution
Wei Tao, Yucheng Zhou, Wenqiang Zhang, Yu Cheng
https://arxiv.org/abs/2403.17927 https:/…
Trackable Agent-based Evolution Models at Wafer Scale
Matthew Andres Moreno, Connor Yang, Emily Dolson, Luis Zaman
https://arxiv.org/abs/2404.10861 https:/…
VCounselor: A Psychological Intervention Chat Agent Based on a Knowledge-Enhanced Large Language Model
H. Zhang, Z. Qiao, H. Wang, B. Duan, J. Yin
https://arxiv.org/abs/2403.13553
Rank-Dependent Predictable Forward Performance Processes
Bahman Angoshtari, Shida Duan
https://arxiv.org/abs/2403.16228 https://arxiv…
This https://arxiv.org/abs/2402.01680 has been replaced.
link: https://scholar.google.com/scholar?q=a
A geometric approach for stability analysis of delay systems: Applications to network dynamics
Shijie Zhou, Wei Lin
https://arxiv.org/abs/2404.18704 https:…
Modeling the Spread of COVID-19 in University Communities
Jeffrey W. Herrmann, Hongjie Liu, Donald K. Milton
https://arxiv.org/abs/2403.10402 https://
NeSy is alive and well: A LLM-driven symbolic approach for better code comment data generation and classification
Hanna Abi Akl
https://arxiv.org/abs/2402.16910
L3GO: Language Agents with Chain-of-3D-Thoughts for Generating Unconventional Objects
Yutaro Yamada, Khyathi Chandu, Yuchen Lin, Jack Hessel, Ilker Yildirim, Yejin Choi
https://arxiv.org/abs/2402.09052
This https://arxiv.org/abs/2310.06155 has been replaced.
initial toot: https://mastoxiv.page/@arXiv_csHC_…
Navigating simplicity and complexity of social-ecological systems through a dialog between dynamical systems and agent-based models
Sonja Radosavljevic, Udita Sanga, Maja Schl\"uter
https://arxiv.org/abs/2402.12086
L3GO: Language Agents with Chain-of-3D-Thoughts for Generating Unconventional Objects
Yutaro Yamada, Khyathi Chandu, Yuchen Lin, Jack Hessel, Ilker Yildirim, Yejin Choi
https://arxiv.org/abs/2402.09052
This https://arxiv.org/abs/2404.11459 has been replaced.
initial toot: https://mastoxiv.page/@arXiv_csCL_…
SONATA: Self-adaptive Evolutionary Framework for Hardware-aware Neural Architecture Search
Halima Bouzidi, Smail Niar, Hamza Ouarnoughi, El-Ghazali Talbi
https://arxiv.org/abs/2402.13204 https://arxiv.org/pdf/2402.13204
arXiv:2402.13204v1 Announce Type: new
Abstract: Recent advancements in Artificial Intelligence (AI), driven by Neural Networks (NN), demand innovative neural architecture designs, particularly within the constrained environments of Internet of Things (IoT) systems, to balance performance and efficiency. HW-aware Neural Architecture Search (HW-aware NAS) emerges as an attractive strategy to automate the design of NN using multi-objective optimization approaches, such as evolutionary algorithms. However, the intricate relationship between NN design parameters and HW-aware NAS optimization objectives remains an underexplored research area, overlooking opportunities to effectively leverage this knowledge to guide the search process accordingly. Furthermore, the large amount of evaluation data produced during the search holds untapped potential for refining the optimization strategy and improving the approximation of the Pareto front. Addressing these issues, we propose SONATA, a self-adaptive evolutionary algorithm for HW-aware NAS. Our method leverages adaptive evolutionary operators guided by the learned importance of NN design parameters. Specifically, through tree-based surrogate models and a Reinforcement Learning agent, we aspire to gather knowledge on 'How' and 'When' to evolve NN architectures. Comprehensive evaluations across various NAS search spaces and hardware devices on the ImageNet-1k dataset have shown the merit of SONATA with up to 0.25% improvement in accuracy and up to 2.42x gains in latency and energy. Our SONATA has seen up to sim$93.6% Pareto dominance over the native NSGA-II, further stipulating the importance of self-adaptive evolution operators in HW-aware NAS.
Language Evolution with Deep Learning
Mathieu Rita, Paul Michel, Rahma Chaabouni, Olivier Pietquin, Emmanuel Dupoux, Florian Strub
https://arxiv.org/abs/2403.11958
SONATA: Self-adaptive Evolutionary Framework for Hardware-aware Neural Architecture Search
Halima Bouzidi, Smail Niar, Hamza Ouarnoughi, El-Ghazali Talbi
https://arxiv.org/abs/2402.13204 https://arxiv.org/pdf/2402.13204
arXiv:2402.13204v1 Announce Type: new
Abstract: Recent advancements in Artificial Intelligence (AI), driven by Neural Networks (NN), demand innovative neural architecture designs, particularly within the constrained environments of Internet of Things (IoT) systems, to balance performance and efficiency. HW-aware Neural Architecture Search (HW-aware NAS) emerges as an attractive strategy to automate the design of NN using multi-objective optimization approaches, such as evolutionary algorithms. However, the intricate relationship between NN design parameters and HW-aware NAS optimization objectives remains an underexplored research area, overlooking opportunities to effectively leverage this knowledge to guide the search process accordingly. Furthermore, the large amount of evaluation data produced during the search holds untapped potential for refining the optimization strategy and improving the approximation of the Pareto front. Addressing these issues, we propose SONATA, a self-adaptive evolutionary algorithm for HW-aware NAS. Our method leverages adaptive evolutionary operators guided by the learned importance of NN design parameters. Specifically, through tree-based surrogate models and a Reinforcement Learning agent, we aspire to gather knowledge on 'How' and 'When' to evolve NN architectures. Comprehensive evaluations across various NAS search spaces and hardware devices on the ImageNet-1k dataset have shown the merit of SONATA with up to 0.25% improvement in accuracy and up to 2.42x gains in latency and energy. Our SONATA has seen up to sim$93.6% Pareto dominance over the native NSGA-II, further stipulating the importance of self-adaptive evolution operators in HW-aware NAS.
An Agent-Based Model of Elephant Crop Raid Dynamics in the Periyar-Agasthyamalai Complex, India
Purathekandy Anjali, Meera Anna Oommen, Martin Wikelski, Deepak N Subramani
https://arxiv.org/abs/2404.09024
Liquid-Graph Time-Constant Network for Multi-Agent Systems Control
Antonio Marino (UR, CNRS), Claudio Pacchierotti (RAINBOW), Paolo Robuffo Giordano (RAINBOW)
https://arxiv.org/abs/2404.13982
This https://arxiv.org/abs/2402.11453 has been replaced.
initial toot: https://mastoxiv.page/@arXiv_csCL_…
A biologically inspired computational trust model for open multi-agent systems which is resilient to trustor population changes
Zoi Lygizou, Dimitris Kalles
https://arxiv.org/abs/2404.10014